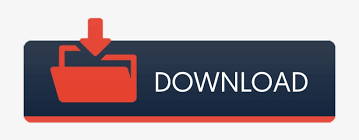
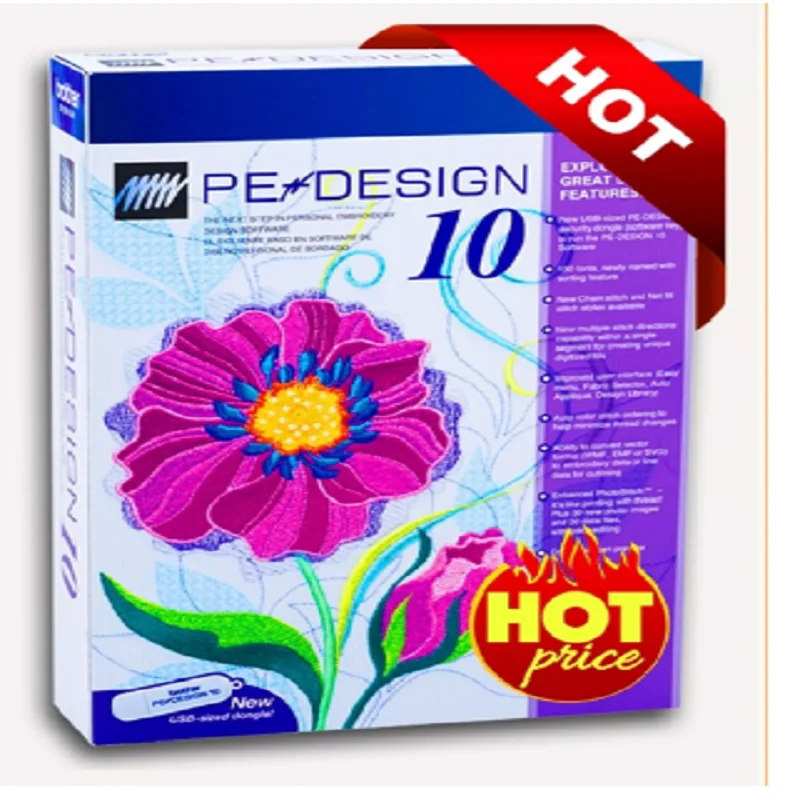
26 investigated the brain areas of mice that mediate the entrance into torpor, showing evidence of which networks of neurons are associated with this process. In the field of natural sciences, many experiments rely on counting biological structures of interest to assess the efficacy of a treatment or the response of an organism to given environmental conditions 26, 27, 28. Counting objects in digital images is a common task for many real-world applications 19, 20, 21, 22 and different approaches have been explored to automate it 9, 10, 23, 24, 25.
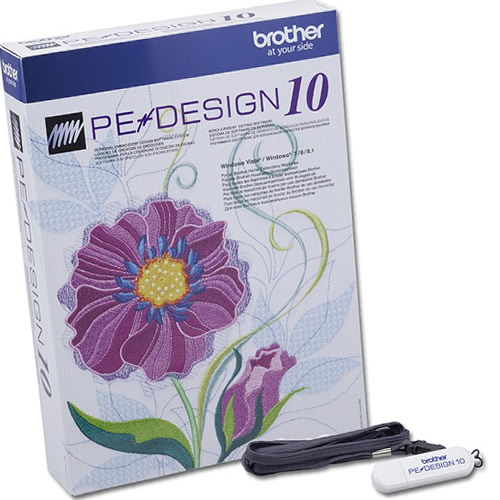
In the wake of this line of applied research, our work tackles the problem of counting cells into fluorescent microscopy pictures.
#Pe design 10 skin
For instance, CNNs have been employed for identification and localization of tumours 7, 8, 9, 10, as well as detection of other structures like lung nodules 11, 12, 13, skin and breast cancer, diabetic foot 14, colon-rectal polyps 15 and more, showing great potential in detecting and classifying biological features 16, 17, 18. Thus, researchers from both academy and industry have started to explore adopting these techniques in fields such as medical imaging and bioinformatics, where the potential impact is vast. Successful examples range from classification and detection of basically any kind of objects 3, 4 to generative models for image reconstruction 5 and super-resolution 6. Finally, we release the pre-trained model and the annotated dataset to foster research in this and related fields.ĭeep Learning models, and in particular Convolutional Neural Networks (CNNs) 1, 2, have shown the ability to outperform the state-of-the-art in many computer vision applications in the past decade. Posterior qualitative assessment by domain experts corroborates previous results, suggesting human-level performance inasmuch even erroneous predictions seem to fall within the limits of operator interpretation. Also, the introduction of weight maps contribute to enhance performances, especially in presence of clumping cells, artifacts and confounding biological structures.

In summary, the c-ResUnet outperforms the competitors with respect to both detection and counting metrics (respectively, \(F_1\) score = 0.81 and MAE = 3.09). In addition, we evaluate through ablation studies the impact of two design choices, (i) artifacts oversampling and (ii) weight maps that penalize the errors on cells boundaries increasingly with overcrowding. Specifically, we introduce a Unet-like architecture, cell ResUnet ( c-ResUnet), and compare its performance against 3 similar architectures. Counts are then retrieved as the number of detected items. We propose a Deep Learning approach that exploits a fully-convolutional network in a binary segmentation fashion to localize the objects of interest. Although such objects are generally easy to identify, the process of manually annotating cells is sometimes subject to fatigue errors and suffers from arbitrariness due to the operator’s interpretation of the borderline cases. Counting cells in fluorescent microscopy is a tedious, time-consuming task that researchers have to accomplish to assess the effects of different experimental conditions on biological structures of interest.
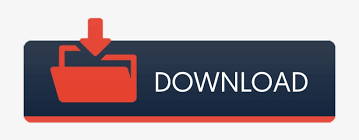